A growing wave of novel substances for performance enhancement or just getting high hits the streets weekly.
On July 12, 2016, EMTs arrived on scene near a subway stop in Brooklyn where something had gone terribly wrong. Multiple people were displaying “zombielike” behavior—lethargy, groaning, and a blank stare. At least 33 had taken an unknown drug, and 18 of them wound up in hospitals (though they were later discharged). Investigators from the NYPD and U.S. Drug Enforcement Agency sent blood and unused samples of the drug, sold as “AK-47 24 Karat Gold,” to Roy Gerona, a clinical chemist at the University of California, San Francisco who does forensic and analytical toxicology work.
Analyzing the mysterious party drug’s chemical signature, Gerona found it identically matched a substance already in his laboratory, one of hundreds of drug-like chemicals his lab had curated into what they call a “prophetic library”—substances they’d synthesized with the expectation of seeing them on the street someday. In less than a day, his lab was able to identify the drug as a chemical called AMB-FUBINACA, a synthetic cannabinoid also known as “Train Wreck 2” that binds to the same receptor as the THC in marijuana—but 85 times as strongly.
“That was a very fast-paced set of events,” Gerona says. “It’s almost like you’re living a movie.”
But identifying designer drugs doesn’t always go so smoothly. Fortunately, a new machine-learning method, published late last year in the journal Nature Machine Intelligence, may help labs with the task of ferreting out the creation of novel drugs designed for recreational users as well as bodybuilders and athletes. The researchers behind the algorithm are also exploring its use in identifying novel performance-enhancing drugs and could be on track to deploy their method in time for 2024’s Olympic games in Paris.
Looking for who knows what
Designer drugs, technically known as new psychoactive substances (NPS), are synthetic chemicals that may not be found in nature and are designed to mimic the effects of traditional illegal drugs and banned substances. They fall into a legal gray area because they are slightly different chemically—enough to raise the question of whether they’re technically banned or not. A new one appears on the market about once per week, and authorities have difficulty identifying them quickly.
Typically, the chemicals or their main ingredients are made in clandestine labs in Asia. Chemists often get ideas from old academic papers. Final synthesis may take place in stash houses in Mexico or the United States. You can buy them on the street or at corner stores or online, depending on their legality. The most common ones mimic drugs like marijuana (including the most famous synthetic cannabinoids, which are sold under names like Spice and K2) or speed and ecstasy (which are sometimes found online and given names like Cloud Nine or Vanilla Sky, collectively known as bath salts). Other new psychoactive substances may mimic traditional psychedelics or opioids.
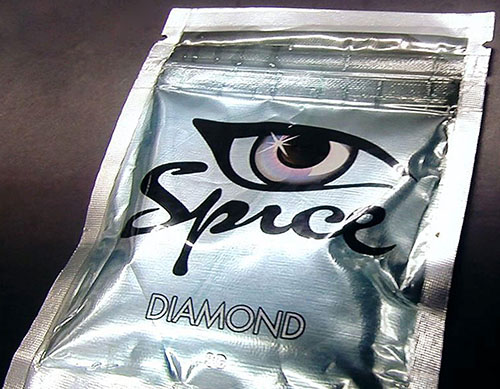
People use them because they believe, often mistakenly, that either they’re legal, hard to detect, or safe. Or maybe they take them because they’re cheaper. Or because they believe they offer a different kind of high, something new to try. Or because they are more available—say at a party in Brooklyn. Sometimes designer drugs are sold, knowingly or unknowingly, as the drugs they mimic.
Gerona first developed his methods, using a technology called liquid chromatography-high resolution mass spectrometry, in 2009, working with a hospital in San Francisco and the San Francisco Poison Control Center. Soon other poison centers in California and around the United States were ringing him up—and then agents from across the whole country. “It just so happened that 2010, when the program was in full swing, coincided with the emergence of bath salts and K2 in the United States,” he says. His current work with the DEA has helped solve close to 700 cases, and he receives 50–60 cases per month. Recently he has also sought the help of the scientists behind the AI method.
Something in a haystack—but it ain’t no needle
Identifying new psychoactive substances is not always straightforward. If a laboratory has a sample of one in its purified form, it could perform an expensive procedure called nuclear magnetic resonance, which uses million-dollar instruments similar to hospital MRIs and is the gold standard for determining a molecule’s chemical structure. But having a pure sample to interrogate is not always the case. Often, the NPS is cut with other substances, sprayed on an herb intended to resemble marijuana, or hidden as a trace component in a blood or urine sample. Nearly all of Gerona’s samples are in the form of blood or urine. To purify the trace drugs inside and analyze them would be time consuming and expensive—and in many cases where the new psychoactive substance is unknown, it is near impossible. You first need to have some idea of what you’re purifying in order to do it.
According to Daniel Pasin, a chemist at the University of Copenhagen and co-author of the new paper applying machine learning to the problem, identifying new psychoactive substances in blood or urine is “like looking for something in a haystack but you don’t know what you’re looking for.” To give some guidance, labs might perform mass spectrometry, identify some suspicious mass measurements in the mix, and predict the associated structures, narrowing the list of needles.
Seeking to streamline this process, Pasin and his colleagues trained a neural network, which they called DarkNPS, to perform the task. The trick was to bias the network to generate realistic structures, chemically similar compounds within the same ballpark of known designer drugs. For this, they relied on a crowdsourced database called HighResNPS, containing the structures and properties of 1,753 known new psychoactive substances.
While that may seem like a lot, it’s really not when it comes to doing this sort of computational chemistry. Generative algorithms sometimes require seeing millions of examples of something before they can produce new realistic examples. The researchers had to make do with fewer than 2,000.
“They make an interesting claim that you could be successful in learning from a small set of data,” says Alexander Tropsha, a computational chemist at the University of North Carolina, who was not involved in the work. “Because of a large diversity of chemicals, you want this training set to be very large, to learn all the hidden rules for making realistic molecules. So that’s what everyone was doing, including my own lab.”
The researchers overcame their paucity of examples using a process called data augmentation. The structure of each molecule in the database is represented as a string of characters called a SMILES (simplified molecular-input line-entry system) string. Different strings can represent the exact same molecule—a cake comes out the same whether the recipe tells you to add the sugar or eggs first—so for each molecule, the researchers produced 100 alternate strings, fleshing out the database.
“We conjecture that our model has developed an implicit understanding of both the chemical scaffolds of known designer drugs and the chemical transformations that are applied to produce novel agents.”
Their neural network was a type used for learning sequences of data, as in language or music. It treated each SMILES string basically like a sentence. Given part of a string, it learned to predict the next character based on what came before. A character represents an atom or bond or the end of a string. Once their AI was trained, it could produce completely new strings, generating one character at a time. (This is roughly how language models such as OpenAI’s GPT-3 write new English prose after trawling Wikipedia.)
The researchers found that the generated structures closely matched the real ones in the database in several ways. For instance, another algorithm predicted that nearly all of them, if synthesized, would pass the blood-brain barrier. The authors write, “we conjecture that our model has developed an implicit understanding of both the chemical scaffolds of known designer drugs and the chemical transformations that are applied to produce novel agents.”
A billion pieces of string
Using their trained neural network, they generated a large universe of 8.9 million unique chemical variants, some of which appeared many times, giving them a total of one billion SMILES strings. They hypothesized that the frequency of any chemical in the set indicated its likelihood of actual manufacture and use. To test this idea, they gathered a set of 194 real new psychoactive substances that had been added to HighResNPS after they’d trained their system. For each, they looked at all the unique strings they’d generated whose mass was within a thousandth of a percent of the real new psychoactive substance’s mass and ranked them by frequency.
“Within about a minute you have a list of hypotheses, and you can just start to flip through them,” says Michael Skinnider, an MD/PhD student at the University of British Columbia in Vancouver and the paper’s primary author. “It’s pretty cool.” And it worked. The actual drug structure was the most frequently predicted 33 percent of the time, and in the top ten 72 percent of the time.
The researchers further honed their method by combining it with an existing algorithm called CFM-ID, which takes a molecular structure and predicts its readout from a sophisticated method called tandem mass spectrometry. Using this approach, the researchers predicted the spectrum for each of their 8.9 million molecules, combined the results with their frequency approach, and found they could correctly identify actual new psychoactive substances 51 percent of the time, and see it in the top ten 86 percent of the time.
What this all means, according to Claude Guillou, a chemist at the European Commission who helps police and customs officers identify new psychoactive substances, is that a system like DarkNPS would be useful, especially for labs that don’t have access to fancy NMR equipment, or that are trying to identify unpurified drugs. He recalls a period a few years ago when new psychoactive substances were popping up twice a week on the street. “So police laboratories and customs authorities were not really prepared for this wave.”
And just as the new machine-learning paper came out at the end of 2021, another new wave of unrecognizable designer drugs started surging.
There’s still a long way to go. Gerona says that the DEA requires confirmed matches, not just a list of candidates, in order to ban a substance or make an arrest, and confirmed matches require testing of both the sample and a known reference substance, which is obviously impossible if the chemical is completely novel. But DarkNPS can still be very useful. It takes time and money to synthesize reference standards. The algorithms might suggest a few possible structures, which human experts can then consider. One or two of these chemicals might look like they’d have effects similar to those seen in people who have taken the unknown substance. The lab might then synthesize only those.
“We hope that something akin to DarkNPS for performance-enhancing drugs is utilized in large sporting events in the future.”
Guillou says a system like DarkNPS will need to be made user friendly. To that end, the researchers have made a web app available to forensic scientists. Skinnider is currently trying to figure out what the credentialing would have to be to keep the tool out of the hands of bad actors. “This information is great in the hands of forensic labs,” he says, “but if you were to distribute it publicly, you might just give the clandestine chemists who make designer drugs a bunch of good new ideas.”
Given a database of performance-enhancing drugs, the same approach might also help identify (or invent) new types of performance-enhancing drugs. Pasin is currently working on it. “We hope that something akin to DarkNPS for performance-enhancing drugs is utilized in large sporting events in the future,” he says. “However, it’s too early to tell.”
The World Anti-Doping Agency, which has an annual budget of about $45 million and handles testing for the Olympics, sees some potential. “Any tool that can help in the identification of new psychoactive substances is useful,” says Olivier Rabin, WADA’s senior director for science and medicine. “AI is certainly a tool we’re interested in. We’ve got several projects that we are running.” He notes that the level of accuracy described in the new paper would not be high enough to sanction an athlete, but the system “may be fine-tuned in the future.” Or at least guide further analysis. The final step, once a new drug is identified, would be to incorporate it into routine anti-doping tests.
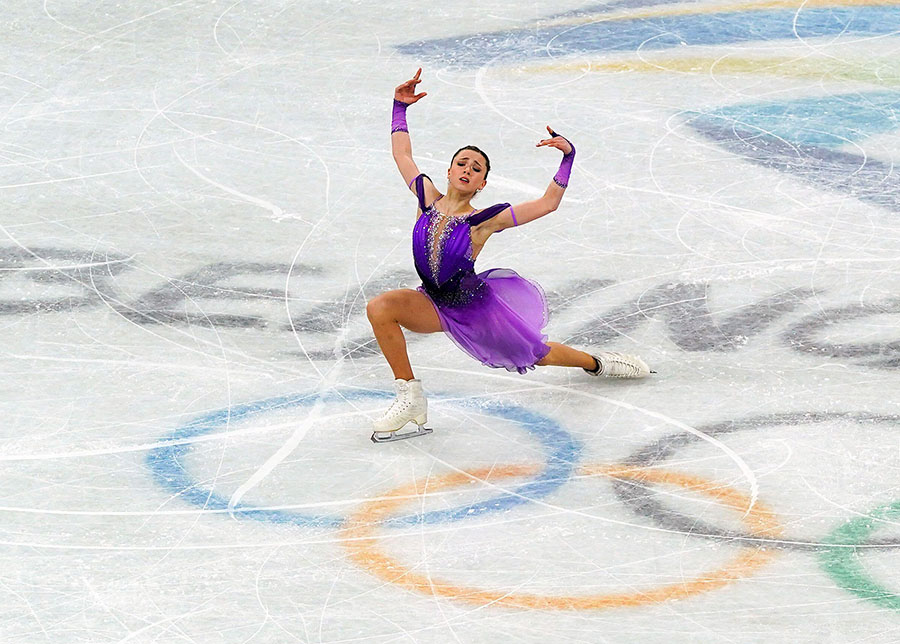
The U.S. Anti-Doping Agency, with an annual budget of around $20 million, tests U.S. athletes at Olympic trials and other competitions (among efforts such as drug education). Urine is considered the gold standard of the work they do because it carries both parent compounds—often anabolic steroids or stimulants—and metabolites for a long time. Chemists analyze it using mass spectrometry.
Matthew Fedoruk, the U.S. Anti-Doping Agency’s chief science officer, says a system like DarkNPS could be useful for identifying performance-enhancing drugs. “With mass spectrometry, you’re kind of flying blind unless you know the structure of the molecule.” A system like DarkNPS could be more helpful.
“Novel substances are something that continually are really important to monitor for us,” says Fedoruk, “as chemists get more fancy with the way they synthesize new molecules.”
But just as tools for catching cheaters become more advanced, so too do the cheaters. It’s a “cat and mouse game,” Fedoruk says—but one where the cats are half blind and the mice are all hopped up on ’roids and speed.
Editor’s note: This story was updated on February 11, 2022 to correct the timeline in the Kamila Valieva photo caption and to clarify the number of unique chemical variants among the one billion SMILES strings.