Workforce diversity, collective oversight, and day-to-day algorithm monitoring are all necessary to mitigate inherent bias.
There’s a joke in Silicon Valley about how AI was developed: Privileged coders were building machine learning algorithms to replace their own doting parents with apps that deliver their meals, drive them to work, automate their shopping, manage their schedules, and tuck them in at bedtime.
As whimsical as that may sound, AI-driven services often target a demographic that mirrors its creators: white, male workers with little time and more disposable income than they know what to do with. “People living in very different circumstances have very different needs and wants that may or may not be helped by this technology,” says Kanta Dihal at the University of Cambridge’s Leverhulme Centre for the Future of Intelligence in England. She is an expert in an emerging effort to decolonize AI by promoting an intersectional definition of intelligent machines that is created for and relevant to a diverse population. Such a shift requires not only diversifying Silicon Valley, but the understanding of AI’s potential, who it stands to help, and how people want to be helped.
Biased algorithms have made headlines in many industries. Apple Health failed to include a menstrual cycle tracking feature until 2015. Leaders at Amazon, where automation reigns supreme, scrapped efforts to harness AI to sift through resumes in 2018 after discovering their algorithm favored men, downvoting resumes with all-female colleges or mentions of women’s sports teams. In 2019, researchers discovered that a popular algorithm used to determine whether medical care was required was racially biased, recommending less care for Black patients than equally ill white patients because it calculated patient risk scores based on individuals’ annual medical costs. Because Black patients had, on average, less access to medical care, their costs were artificially low, and the algorithm faultily associated Blackness with less need for medical treatment.
Industries like banking have been more successful than others in implementing equitable AI (think: creating new standards for the use of AI and developing systems of internal checks and balances against bias), according to Anjana Susarla, the Omura-Saxena Professor in Responsible AI at Michigan State University. Health care and criminal justice, where algorithms have shown racial bias in predicting recidivism, are more troubling examples. Susarla says decolonizing AI will require the collective oversight of boards of directors, legislators, and regulators, not to mention those who implement and monitor the day-to-day outcomes of AI in every industry—not just the techies behind the tools.
“You have to have some separation between the person who created the AI and the person who can take some responsibility for the consequences that will arise when they are implementing it,” Susarla says.
The myth of the lone genius
Science fiction films and TV shows and even stock images portray AI as white, plastic robots, often created by a white male protagonist. (Try a Google search for “intelligent machines” to test this.) Instead of showing legions of engineers and developers behind the scenes working toward new technologies, Dihal says tech heroes are painted as workaholic loner-heroes.
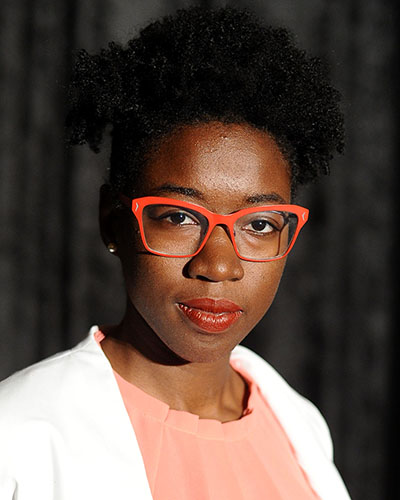
In a 2020 study, Dihal and her University of Cambridge co-author Stephen Cave found that these media portrayals perpetuate racial stereotypes, threaten to position white robots above people of color, and situate AI in the public consciousness as a white-collar issue. As AI touches nearly every facet of our society, decolonizing it will require widespread changes to everyday narratives, including fictional ones, as well as the tech itself.
When it comes to tasks like content moderation or image recognition, “people often don’t know how many hours of manual labor have gone into it, or how many hours are still going into it,” Dihal says. Instead, users wrongly attribute unseen human labor to intelligent machines.
But tech giants require huge workforces, and often “reinforce or reawaken colonial power structures” in the process of engaging their workforces, Dihal continues. She points to tech leaders who employ legions of low-paid “click workers” in India, China, or the United States, where they complete micro tasks like labeling or verifying images online to train AI algorithms. The work is low-paid, often below minimum wage, and can be sporadic, with micro tasks popping online at odd hours, according to online reviewers of Clickworker, a firm that matches online workers with these tasks. Companies like Clickworker promise work-from-home freedom, though a 2017 study found clickworkers netted just $3.31 per hour.
There are also people, like delivery workers, who are part of AI whether they like it or not. “Those people don’t have power or don’t have the authority to either buck the system or not be part of it,” says Amber Thompson, founder of de-bias, an AI-driven public review platform that measures business outcomes against a range of social issues.
Higher-paid workers, like software developers, software engineers, and UX (user experience) engineers may come from all over the world, Dihal says. “But still, in the C-suite, higher up in the pipeline, the gender balance and the ethnicity balance very quickly skew toward white male.”
AI needs a human touch
Fortunately, for now, an all-knowing AI that can efficiently solve problems without human intervention is more media construct than reality, according to Dihal. AI needs human oversight.
“Efficiency is very important, but efficiency means cutting corners,” says Susarla, who consults with companies implementing AI. When the focus is solely on tactical performance, she says, “we should understand that it’s not always going to make things better.” Sometimes, simple algorithms yield the most accurate AI.
“There will always be bias. De-biasing is the process to mitigate those biases before they happen.”
But merely replacing white software developers with techies of color isn’t a fix, according to Thompson. “If your actual process doesn’t mean you have to listen, respond, or include [people], then it’s the same thing only it’s worse because now you’ve tokenized people and you’ve probably created a more biased product.”
At de-bias, that means gathering data about the impacts of a company’s actions from leadership, employees, board members, community members, and other stakeholders, then creating a rubric to measure equity efforts, employee wellbeing (everything from health insurance to homeownership), community development, advocacy efforts, and more, with an eye to change. “There will always be bias,” Thompson says. “De-biasing is the process to mitigate those biases before they happen.” Like so much of decolonizing AI, it requires a ton of input from a wide range of people.
Thompson says truly decolonized AI is impossible without collective action to topple tech giants like Google or Twitter, particularly when shareholder ROI has so much sway, so she’s currently focusing de-bias on smaller startups. Susarla hopes that a combination of compliance with existing civil rights legislation, new governmental regulations, and corporate self-governance (think: boards of directors that provide oversight to AI initiatives, gauging outcomes against the company’s mission and values) will aid AI’s evolution.
Ultimately, Susarla says, equitable AI is explainable. It’s not a white robot that mysteriously announces its findings. If an algorithm determines you’re not eligible for a mortgage, a loan officer should be able to explain why. If a stack of resumes never makes it to HR, hiring agents should be able to articulate how candidates are evaluated.
“How much does a person have to change themselves to function with technological systems that increasingly govern our lives?” wrote computer scientist and poet Joy Buolamwini in a 2019 Time editorial. Buolamwini founded the Algorithmic Justice League to spotlight and rectify bias in facial recognition algorithms. In a world increasingly laced with AI everything, it will take a growing number of voices—and especially those from marginalized groups—to determine when, how, and whether we correct it.