Machine Learning Illuminates the Body’s Dark Matter
A startup finds patterns that experts in personalized medicine say they couldn’t see before.
When it comes to using computers to analyze medical data, it takes a lot to impress Larry Smarr.
Smarr, a computer scientist at a UC San Diego/UC Irvine research institute called Calit2, is a pioneer of the quantified-self movement. He regularly analyzes his blood and stool for 150 biomarkers. If he notices something odd, he’ll adjust his daily activity, whether that means taking a few extra laps around campus to burn more calories or tweaking the strain of probiotic he’s supplementing with. Thanks to all this data, a few years ago he realized he had Crohn’s disease before his doctor did—and when he eventually needed surgery for the condition, he supplied his surgeon with a 3-D model of his colon so she could familiarize herself with the layout before the operation.
Because Smarr is so well-versed in amassing and analyzing medical data, it was striking to hear that a new machine-learning startup, Pattern Computer, had something new to show him.
One of Smarr’s obsessions is the interplay of bacteria in our gut—our microbiome—and how little we know about it. (“The dark matter of the body,” he calls it.) Smarr is trying to figure out which shifts and fluctuations in the microbiome are normal, and which might cause disease. To do that, he and colleagues compared the gut bacteria of healthy people to the microbiomes of people with various stages of inflammatory bowel disease. Even with only 62 people providing samples in this study, it generated a massive amount of data, because the bacteria in our guts produce about 10,000 different kinds of proteins that each perform a different biological function. And the relative abundance of these protein families varied in each of the human subjects.
It’s been known that a certain subset of these protein families is less common in people with IBD than in healthy people. But when Smarr gave Pattern Computer that data, it was able to narrow down the subset substantially. It turned out that nine of those 10,000 protein families appeared to be the most associated with inflammatory bowel disease. Zeroing in on those nine and their biochemical pathways could offer new clues about the ways our microbiome affects our health and open up new interventions.
“That was really a discovery,” Smarr says. After looking at this data for years with a variety of software tools, he had not noticed “the pattern that was blocked inside of this big mess of data points.”
That’s Pattern Computer’s promise: that its machine-learning system can find correlations that other systems can’t, even when it hasn’t been told what to look for. The company is led by tech forecaster Mark Anderson and launched this week with around $6 million in funding and clients that range from “companies to countries,” he says. With time, it’ll move into different industries, Anderson adds, but right now it’s focusing on biomedicine.
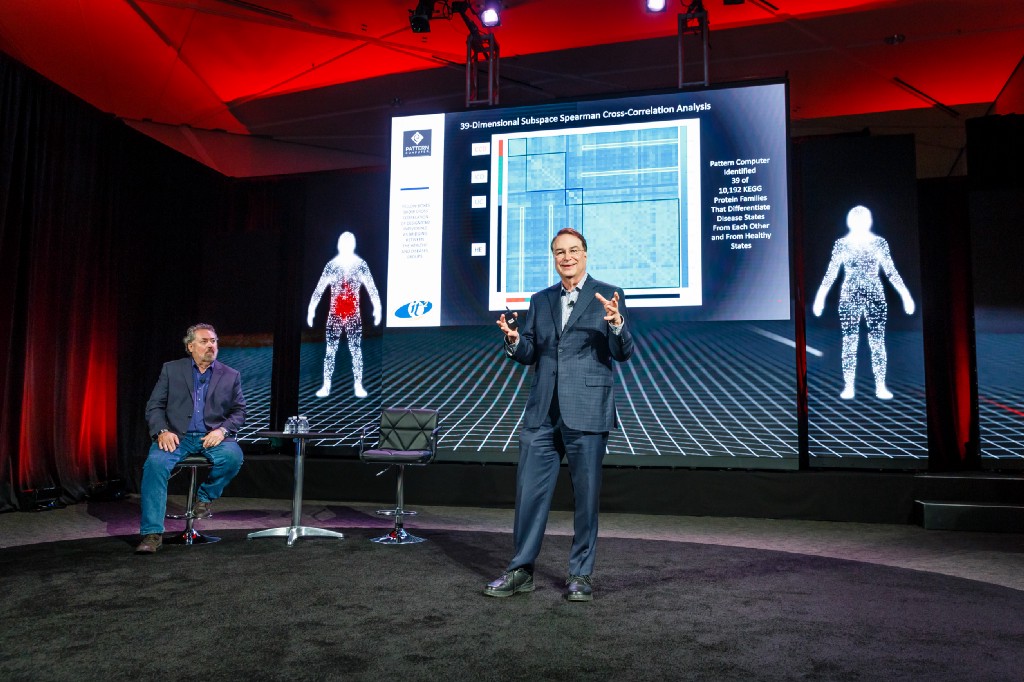
It has a lot of competition. Medical researchers, pharmaceutical companies, hospitals, and doctors are trying a wide range of advanced computing techniques to try to find insights in data. What’s especially tricky is that this data comes in many forms, from the quantities in blood tests to the readouts of genomes to the text in journal articles and patient records. Neither Pattern Computer nor any other single company or algorithm is likely to master all of it. IBM has shown as much. Despite lots of hype about how its Watson technologies—based on the system that won on Jeopardy!—are being used at Memorial Sloan Kettering Cancer Center and other hospitals, they’ve done little to change how doctors diagnose and treat disease.
Progress in using machine learning in medicine is instead coming in smaller steps. Ben Brown, a computational biologist at Lawrence Berkeley National Laboratory, is trying to discover how interactions between genes lead to breast cancer. But because there is such wide variation in breast cancer tumors, it’s impossible for us to study all the relevant gene interactions “by brute force,” Brown says. He has used Pattern Computer’s technology to find a three-way gene interaction that is correlated with low cancer survival rates. Follow-up research could explore targeted therapies for that interaction.
Higher resolution
In 2009, Leroy Hood, one of the developers of gene-sequencing technology and a trailblazer for a comprehensive view of health called “systems biology,” wrote an article for Newsweek called “A Doctor’s Vision of the Future of Medicine.” In the future he imagined, people were testing their own blood with at-home devices, allowing their doctors to analyze mind-boggling amounts of information about their health, down to the level of genes. That article was set in … June 2018.
We’re not where Hood envisioned we’d be by now, because only part of the equation has arrived. “We have enormous amounts of data,” Hood says. “We’re just beginning to scratch the surface of what we can do with it.”
Through a health-coaching service called Arivale and other research, Hood is devoted to bringing about what he calls P4 medicine: predictive, preventive, personalized, and participatory. The challenge, as he put it, is to analyze data of many different types. As an example, he pointed to a P4 pilot study on 108 of his friends. For nine months, his team gathered information on them by sequencing their genome, taking regular blood and stool tests, and giving them self-trackers for their sleep and physical activity. To fully make sense of the data and pull several actionable suggestions from it, Hood says he’ll need more tools like Pattern Computer’s.
Smarr notes that the breakthrough Pattern Computer made in his research is just “one experiment.” To be sure, the startup might prove to play a small role in the advance of data-driven medicine. But Hood says he’s optimistic that P4 could be a reality before long. Finally, he says, researchers can “look at data and disease with a resolution we’ve never had before.”