AI can help overcome both scientific and financial hurdles to tackle this serious threat to global health.
Imagine a simple scratch becoming a life-threatening illness, the way it used to be decades ago. Well, that could happen again. The future threatens emerging outbreaks as bacteria become increasingly resistant to the antibiotics normally used to counter run-of-the-mill infections. But the future could also offer a powerful potential solution in the battle against antimicrobial resistance: artificial intelligence.
The World Health Organization (WHO) has identified antimicrobial resistance as one of the top 10 threats to global health. Its concern reflects the fact that antibiotics are not only important to counter routine infections but also for protecting people undergoing any number of clinical procedures that involve a risk of infection, such as dialysis and organ transplants.
The WHO estimates that 700,000 people die of antimicrobial-resistant infections each year and that number could balloon to 10 million a year by 2050 unless new alternatives emerge or a way is found to overcome the threat posed to the efficacy of antibiotics, long hailed as one of modern medicine’s most consequential discoveries.
Thanks to innovative research at a number of universities and at least one private company, scientists and clinicians are optimistic that AI may hold the key to counteracting the threat posed by antimicrobial resistance, which experts often refer to simply as AMR.
“AI can be a game changer in the fight against AMR by enabling the rapid and accurate detection of resistance, favoring improved antibiotic use and maximizing patient outcomes,” says Alexandre Drouin, an adjunct professor of computer science at Laval University in Quebec City, Canada. “It also holds the promise of uncovering new, unsuspected drivers of AMR, which may serve to develop the next generation of antimicrobials.”
One of the major concerns of antimicrobial resistance is that clinicians are running out of alternatives for many bacterial infections. The last truly novel class of antibiotics were discovered in the 1980s. Since then, antibiotic discovery has stalled, leading to what scientists call a discovery void since the late 1980s, with no new antibiotics in the market over the past 35 years.
A new antibiotic would have to earn at least $300 million per year in U.S. sales—but most earn between $15–$50 million per year.
Globally, the financial incentive for new discovery simply may not exist because most antibiotics are taken by people in low-income settings. Even in the United States, the most lucrative drug market in the world, it’s unlikely that any new antibiotic would ever achieve “blockbuster” status—a term used to describe a drug that generates at least $1 billion in annual sales. Blockbuster drugs tend to be either extremely high-priced biologics—like antibody treatments for cancer and autoimmune diseases—or far less expensive drugs that are taken by many, many people every single day for years and years to treat chronic conditions like high cholesterol, blood pressure, or depression. Lots of people in the United States take antibiotics, but usually only for a week or two at the most.
The journal Nature reported in 2020 that by one estimate, a new antibiotic would have to earn at least $300 million per year in U.S. sales to be considered a sustainable investment. But none of the antibiotics currently on the American market achieve such returns. Only a few see annual sales above $100 million, and most earn between $15–$50 million per year.
The AI movement asks: Given that those earnings are not likely to change, is it possible to incentivize developing new antibiotics by making them cheaper to discover?
“Currently it is estimated that to develop a drug you need over $2 billion,” says César de la Fuente, an assistant professor of psychiatry who heads the machine biology group at the University of Pennsylvania. “AI can help accelerate the discovery process, dramatically reducing associated costs.”
“By using machines, the timescale required for discovery of interesting molecules is compressed substantially. Instead of having to wait months to years to discover something new, we can do it in days to weeks,” de la Fuente adds.
AI can reduce the risk of failure and the financial costs associated with the antibiotic-discovery pipeline needing exhaustive experimental screening of natural substances for antibiotic properties. “Digital discovery efforts can yield many more molecules of interest, thus providing a higher number of preclinical candidates with the potential to enter the clinic,” de la Fuente says.
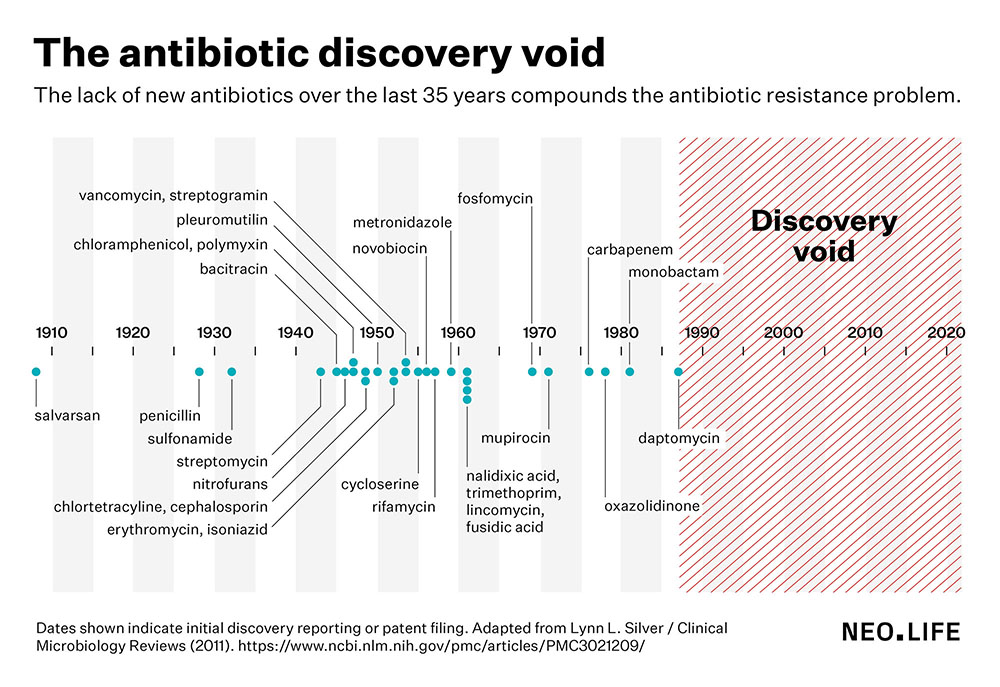
Resistance is future
If we could abstract the awful human tragedy from deadly antibiotic resistance and look at it from a strictly scientific perspective, it’s actually a fascinating phenomenon—like seeing evolution on display. It occurs naturally all the time in harmless soil-dwelling bacteria that can develop resistance as they protect themselves from harmful substances naturally found in the environment. However, the resistance becomes problematic, even dangerous, when those same bacteria find their way into the food stream or clinical settings and cause infections that are resistant to antibiotics.
Overuse and misuse of antibiotics in humans, animals, and plants can hasten the evolution of resistance as the bacteria adapt to survive. According to the U.S. Centers for Disease Control and Prevention, between a third and a half of antibiotics used in hospitals are inappropriately or unnecessarily prescribed. That’s because growth-based antimicrobial susceptibility tests, the current gold-standard method for diagnosing bacterial infection and antibiotic resistance, are slow. They typically require 18–24 hours before they produce a result. And clinicians, doing their best to treat infections fast before the bacteria can do irreparable damage, often prescribe broad-spectrum antibiotics that target numerous types of bacteria even before they have identified the specific infection they are dealing with.
Speedier diagnosis of infection would dramatically lower the risk of bacteria developing antimicrobial resistance due to antibiotic misuse—and that is where artificial intelligence comes in. Scientists such as Timothy Inglis, associate professor at the University of Western Australia in Perth have begun using AI to improve the speed and accuracy of antimicrobial susceptibility testing.
By combining a high-tech benchtop flow cytometer antimicrobial susceptibility testing method with an AI method known as supervised machine learning, Inglis and his colleagues have been able to dramatically accelerate the process, confirming bacterial infections and identifying the most suitable antibiotic to treat them in less than three hours. Inglis’s method has yet to be implemented in clinical laboratories for field-testing, but he is confident that it holds promise for fully automated rapid antibiotic resistance diagnosis.
“At a cell biology level, [antimicrobial resistance] is a wicked problem”, Inglis says. However, he adds, “We have shown that its complexity can be reduced by smart application of machine learning tools.”
The DNA of AMR
All the information needed to identify antibiotic resistance is embedded in a bacteria’s genome or DNA. Extracting the data, however, is a needle-in-the haystack process because it requires an efficient scan of potentially millions of changes in bacterial genomes.
At Laval University, Drouin has used other AI systems known as classification and regression trees and set covering machines to generate 107 highly accurate models of antibiotic resistance covering 12 human bacteria and 56 different antibiotics from whole bacterial genomes.
“Our algorithms find small snippets of the bacterial genomes, called k-mers, for which the presence or absence is predictive of AMR”, Drouin says. “This could arise, for example, if a specific snippet is present in all resistant bacterial isolates but [not] in the bacteria that are sensitive to antimicrobials.” For instance, one of his models predicted resistance of the tuberculosis-causing bacterium to the powerful antibiotic-of-last-resort kanamycin with 93.7 percent accuracy using two snippets located in genes known to be associated with antimicrobial resistance.
“Our AI methods optimize for accurate prediction of AMR,” Drouin says—something that could help speed identification of infections. “Ultimately, these may serve to develop rapid and cost-effective diagnostic tests that may end up in hospital laboratories.”
They also may help develop new drugs against emerging infections that are resistant to existing drugs. The prediction models devised by Drouin promise to be able to quickly identify unknown genomic regions of bacteria that indicate antibiotic resistance. An example would be an AI-identified link between a change in a specific gene of pneumonia-causing bacteria and its resistance to the antibiotic erythromycin. These newly identified elements of bacteria, when validated in biological experiments, could be used to develop new antimicrobial drugs.
While Drouin is mining bacterial genomes for information on antimicrobial resistance, scientists at the University of Pennsylvania have found that knowledge to counter antibiotic resistance is present in our own genomes in the form of antimicrobial human proteins. De la Fuente is using artificial intelligence to scan the entire human proteome for antibiotic discovery. His team has identified several small clusters of proteins that were effective as antibiotics in animal models.
“These days we are generating enormous amounts of data, both clinical and on AMR, and we need the help of AI to make sense of it all.”
“I think of biology as an information source,” de la Fuente says. “Everything can be thought of as a code. Proteins are driven by an amino acid code and DNA by a nucleotide code. What if we could develop computational methods to explore this code to find new drugs? This is what we have done already by performing the first proteome-wide exploration of the human body as a source of antibiotics.”
AI is also helping scientists predict resistance evolution patterns: In other words, the evolution of bacteria from antibiotic-sensitive to antibiotic-resistant strains. If all goes well, this data could help clinicians decide which specific antibiotic treatment is most suitable to slow, if not stop, the spread of antimicrobial resistance in a particular person. That would prevent them having to prescribe broad-spectrum antibiotics that could drive resistance.
“These days we are generating enormous amounts of data, both clinical and on AMR, and we need the help of AI to make sense of it all,” Pablo Catalán, assistant professor of mathematics at Charles III University of Madrid in Spain, says via email. “AI methods can give new perspectives to inform experts of their clinical decisions.”
Catching the wave
Among the most useful types of data being generated in clinical laboratories to expand the fight against antimicrobial resistance is minimum inhibitory concentration, commonly known as MIC. The term refers to the lowest amount of antibiotic needed to inhibit the growth of a specific type of bacteria. The most effective antibiotics are those for which bacteria have a low MIC value, meaning that it takes less of the antibiotic to knock it down. The higher the MIC value for an antibiotic-bacteria pairing, the higher the resistance of bacteria to the tested antibiotic, the more drugs you have to administer, and the greater the odds resistance will emerge.
“If you plot MIC distributions [across all patients] over time, you will see a wave that seems to move coherently in most pairs of bacteria and antibiotics,” Catalan says. “Using AI methods, we can try to predict how that wave will move in the future. For some pathogens that are susceptible to one antibiotic, we can clearly see that this wave is getting closer to the clinical breakpoint for determining resistance. Which means that, if the trend continues, the bacteria will reach clinical resistance.”
He adds that the predictive model produced by artificial intelligence “helps antibiotic stewardship in the sense that we can learn from the data what kind of pathogens are gaining resistance to some antibiotics. And so, we should change our therapies to better fit what we see.” Catalán notes that AI can also help doctors decide on new combination therapies or sequential therapies when they know which antibiotics a person was prescribed and whether the treatment was successful.
Detecting a valid bacterial resistance marker doesn’t mean you’ve identified a valid new drug target unless you can prove that experimentally.
Drouin sees potential shortcomings unless researchers can draw on other scientific disciplines such as biochemistry to make sense of the mutations they find. “While these AI-based models hold the promise of deepening our understanding of AMR, these results must be interpreted with caution,” he says. “The reason is that our algorithms can find regions that are statistically associated with AMR, but not necessarily its true causes.”
For instance, one bacterial strain could be resistant to two different antibiotics, and there could be a single set of genetic markers that accurately predict its resistance to both drugs. But that wouldn’t tell you why the bacteria resist the two drugs—or even whether the mechanisms of resistance are the same. Nor would it reveal if the markers are even linked to the resistance in a causal way. Without knowing those details, there’s only so far you can go in designing new drugs. So in other words, simply detecting a valid bacterial resistance marker doesn’t mean you’ve identified a valid new drug target unless you can prove that experimentally.
Despite such reservations, de la Fuente is excited by AI’s promise as a means of developing antibiotics that can save lives without fear of antimicrobial resistance. Applying AI to antibiotic research, known as digital antibiotic discovery, he says, “is a very young and emerging field.”
“It was not even a field—even five years ago,” de la Fuente says. “Therefore, we are still learning how to do things, pushing the boundaries, learning a lot.”